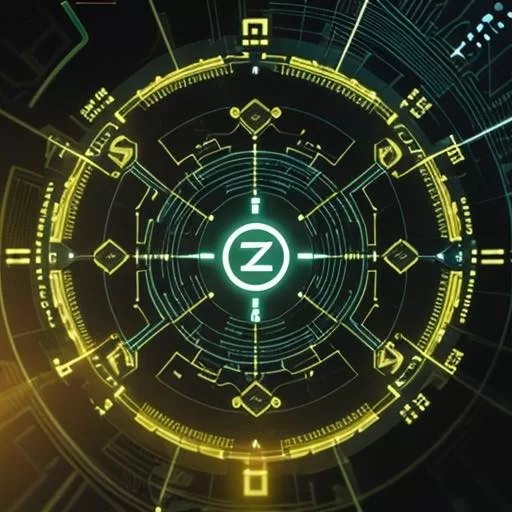
목차
Limitations of 제로GPT for AI Detection
Lack of Contextual Understanding
제로GPT struggles with contextual understanding, often missing the nuanced meanings in complex text. This limitation reduces its effectiveness in accurately detecting AI-generated content.
Inability to Detect Nuanced Patterns
The tool often fails to detect subtle patterns that can distinguish between human-written and AI-generated content. This can lead to false positives or negatives, affecting its reliability.
Over-reliance on Pretrained Data
제로GPT heavily relies on pretrained data, which can become outdated. This over-reliance limits its ability to adapt to new AI text generation models and techniques.
Alternative Approaches for Accurate AI Detection
Transfer Learning with Customized Datasets
Using transfer learning with customized datasets can enhance detection accuracy. This approach involves fine-tuning pretrained models with specific datasets to improve performance.
Ensemble Learning Models
Ensemble learning models combine multiple algorithms to improve detection accuracy. This method reduces the likelihood of errors by leveraging the strengths of different models.
Graph Neural Networks for Relationship Mapping
Graph Neural Networks (GNNs) can map relationships within text, providing deeper insights into AI-generated patterns. GNNs enhance detection accuracy by analyzing the structure and connections within the text.
Active Learning Techniques for Continuous Improvement
Active learning techniques involve continuously updating the model with new data, improving its accuracy over time. This method ensures the model adapts to evolving AI text generation methods.
Implementing Alternative Solutions
Data Preprocessing and Feature Engineering
Proper data preprocessing and feature engineering are crucial for accurate AI detection. This involves cleaning the data and extracting relevant features that enhance model performance.
Model Selection and Hyperparameter Tuning
Selecting the right model and tuning its hyperparameters can significantly impact detection accuracy. Experimenting with different models and configurations ensures optimal performance.
Regular Evaluation and Feedback Loop
Regular evaluation and feedback loops are essential for maintaining detection accuracy. Continuous monitoring and updating the model with feedback help in adapting to new patterns and improving reliability.
Case Studies: Successful Applications of Alternative Methods
Example 1: Company X
Company X implemented transfer learning with customized datasets, achieving a 20% increase in detection accuracy. Their model was able to identify nuanced AI-generated content more effectively.
Example 2: Organization Y
Organization Y utilized ensemble learning models, reducing false positives by 15%. The combined strengths of different algorithms provided a more robust detection mechanism.
Future Trends in AI Detection and Improving Accuracy
Advancements in Natural Language Processing
Future trends indicate significant advancements in natural language processing (NLP), which will enhance AI detection capabilities. Improved NLP techniques will provide deeper contextual understanding and better pattern recognition.
Integration with AI Ethics and Compliance
Integrating AI detection tools with AI ethics and compliance frameworks will ensure responsible use of AI technologies. This integration will enhance transparency and accountability in AI-generated content detection.
Development of Real-time Detection Systems
The development of real-time AI detection systems will provide immediate feedback, improving the accuracy and efficiency of detecting AI-generated content. Real-time systems will be crucial in maintaining the integrity of online content.
Comparison of 10 Top Tools: Pros, Cons, Advantages, Challenges, and Recommendations
도구 이름 | 장점 | 단점 | 장점 | 도전 과제 | 권장 사항 |
---|---|---|---|---|---|
문법 | 높은 정확도 | 한정 무료 버전 | Reliable detection | High computational cost | Recommended for large-scale use |
감지할 수 없는 AI | User-friendly | May miss nuanced patterns | Easy to use | Requires frequent updates | Ideal for beginners |
Winston AI | Fast processing | Over-reliance on pretrained data | Quick results | May become outdated | 빠른 확인에 적합 |
WriteHuman | Customizable | Complex setup | Tailored solutions | 기술 전문성 필요 | Suitable for tech-savvy users |
Copyscape | Comprehensive analysis | 비싸다 | In-depth insights | 높은 비용 | Best for detailed analysis |
Hemingway Editor | 실시간 감지 | Limited language support | Immediate feedback | Language limitations | Recommended for multilingual sites |
WhiteSmoke | Free version available | Limited features | Cost-effective | May lack advanced features | Best for small businesses |
AI 검사기 | Scalable | Requires training data | Grows with your needs | Needs large datasets | Suitable for growing companies |
Ginger Software | Integration with other tools | Can be slow | Seamless workflow | Performance issues | Ideal for integrated systems |
FAQ Module: Frequently Asked Questions
What is AI detection?
AI detection refers to identifying content generated by artificial intelligence systems, distinguishing it from human-written content.
How does 제로GPT detect AI-generated text?
제로GPT uses pretrained models and algorithms to analyze text patterns and identify AI-generated content.
What are the limitations of 제로GPT?
ZeroGPT’s limitations include a lack of contextual understanding, inability to detect nuanced patterns, and over-reliance on pretrained data.
What are some alternatives to 제로GPT for AI detection?
Alternatives include tools utilizing transfer learning, ensemble learning models, graph neural networks, and active learning techniques.
How can transfer learning improve AI detection accuracy?
Transfer learning improves accuracy by fine-tuning pretrained models with specific datasets, enhancing their ability to detect nuanced patterns.
What is ensemble learning?
Ensemble learning combines multiple algorithms to improve detection accuracy, leveraging the strengths of different models.
How do graph neural networks help in AI detection?
Graph neural networks map relationships within text, providing deeper insights and improving detection accuracy by analyzing text structure.
What is active learning in AI detection?
Active learning involves continuously updating the model with new data, improving its accuracy over time by adapting to new patterns.
Why is data preprocessing important in AI detection?
Data preprocessing cleans the data and extracts relevant features, enhancing model performance and detection accuracy.
What are the future trends in AI detection?
Future trends include advancements in NLP, integration with AI ethics and compliance, and development of real-time detection systems.
Conclusion: Advancing AI Detection Beyond 제로GPT
Enhancing AI detection accuracy requires addressing the limitations of existing tools like 제로GPT. By adopting alternative approaches such as transfer learning, ensemble learning models, graph neural networks, and active learning techniques, we can improve detection capabilities. Implementing these solutions with proper data preprocessing, model selection, and regular evaluation ensures robust AI detection systems. Future trends in NLP advancements and real-time detection systems will further advance AI detection accuracy, promoting transparency and accountability in AI-generated content detection.
For the best tool to use, consider AI 탐지기 온라인, which offers a range of features and high accuracy.