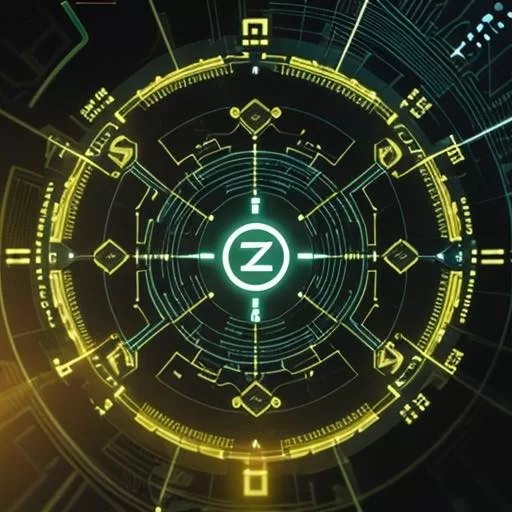
Daftar Isi
Limitations of ZeroGPT for AI Detection
Lack of Contextual Understanding
ZeroGPT struggles with contextual understanding, often missing the nuanced meanings in complex text. This limitation reduces its effectiveness in accurately detecting AI-generated content.
Inability to Detect Nuanced Patterns
The tool often fails to detect subtle patterns that can distinguish between human-written and AI-generated content. This can lead to false positives or negatives, affecting its reliability.
Over-reliance on Pretrained Data
ZeroGPT heavily relies on pretrained data, which can become outdated. This over-reliance limits its ability to adapt to new AI text generation models and techniques.
Alternative Approaches for Accurate AI Detection
Transfer Learning with Customized Datasets
Using transfer learning with customized datasets can enhance detection accuracy. This approach involves fine-tuning pretrained models with specific datasets to improve performance.
Ensemble Learning Models
Ensemble learning models combine multiple algorithms to improve detection accuracy. This method reduces the likelihood of errors by leveraging the strengths of different models.
Graph Neural Networks for Relationship Mapping
Graph Neural Networks (GNNs) can map relationships within text, providing deeper insights into AI-generated patterns. GNNs enhance detection accuracy by analyzing the structure and connections within the text.
Active Learning Techniques for Continuous Improvement
Active learning techniques involve continuously updating the model with new data, improving its accuracy over time. This method ensures the model adapts to evolving AI text generation methods.
Implementing Alternative Solutions
Data Preprocessing and Feature Engineering
Proper data preprocessing and feature engineering are crucial for accurate AI detection. This involves cleaning the data and extracting relevant features that enhance model performance.
Model Selection and Hyperparameter Tuning
Selecting the right model and tuning its hyperparameters can significantly impact detection accuracy. Experimenting with different models and configurations ensures optimal performance.
Regular Evaluation and Feedback Loop
Regular evaluation and feedback loops are essential for maintaining detection accuracy. Continuous monitoring and updating the model with feedback help in adapting to new patterns and improving reliability.
Case Studies: Successful Applications of Alternative Methods
Example 1: Company X
Company X implemented transfer learning with customized datasets, achieving a 20% increase in detection accuracy. Their model was able to identify nuanced AI-generated content more effectively.
Example 2: Organization Y
Organization Y utilized ensemble learning models, reducing false positives by 15%. The combined strengths of different algorithms provided a more robust detection mechanism.
Future Trends in AI Detection and Improving Accuracy
Advancements in Natural Language Processing
Future trends indicate significant advancements in natural language processing (NLP), which will enhance AI detection capabilities. Improved NLP techniques will provide deeper contextual understanding and better pattern recognition.
Integration with AI Ethics and Compliance
Mengintegrasikan alat pendeteksi AI dengan kerangka kerja etika dan kepatuhan AI akan memastikan penggunaan teknologi AI yang bertanggung jawab. Integrasi ini akan meningkatkan transparansi dan akuntabilitas dalam pendeteksian konten yang dihasilkan oleh AI.
Development of Real-time Detection Systems
Pengembangan sistem deteksi AI secara real-time akan memberikan umpan balik langsung, meningkatkan akurasi dan efisiensi dalam mendeteksi konten yang dihasilkan oleh AI. Sistem waktu nyata akan sangat penting dalam menjaga integritas konten online.
Comparison of 10 Top Tools: Pros, Cons, Advantages, Challenges, and Recommendations
Nama Alat | Kelebihan | Kekurangan | Keuntungan | Tantangan | Rekomendasi |
---|---|---|---|---|---|
Grammarly | Akurasi tinggi | Versi gratis terbatas | Deteksi yang andal | Biaya komputasi yang tinggi | Direkomendasikan untuk penggunaan skala besar |
AI yang tidak terdeteksi | Ramah pengguna | Mungkin melewatkan pola yang bernuansa | Mudah digunakan | Membutuhkan pembaruan yang sering | Ideal untuk pemula |
Winston AI | Pemrosesan cepat | Terlalu bergantung pada data yang sudah terlatih | Hasil cepat | Dapat menjadi usang | Terbaik untuk pemeriksaan cepat |
WriteHuman | Dapat disesuaikan | Penyiapan yang rumit | Solusi yang disesuaikan | Membutuhkan keahlian teknis | Cocok untuk pengguna yang paham teknologi |
Copyscape | Analisis komprehensif | Mahal | Wawasan mendalam | Biaya tinggi | Terbaik untuk analisis terperinci |
Editor Hemingway | Deteksi waktu nyata | Dukungan bahasa yang terbatas | Umpan balik langsung | Keterbatasan bahasa | Direkomendasikan untuk situs multibahasa |
WhiteSmoke | Tersedia versi gratis | Fitur terbatas | Hemat biaya | Mungkin kekurangan fitur-fitur canggih | Terbaik untuk bisnis kecil |
Pemeriksa AI | Terukur | Membutuhkan data pelatihan | Tumbuh bersama kebutuhan Anda | Membutuhkan set data yang besar | Cocok untuk perusahaan yang sedang berkembang |
Perangkat Lunak Ginger | Integrasi dengan alat lain | Bisa lambat | Alur kerja yang mulus | Masalah kinerja | Ideal untuk sistem terintegrasi |
FAQ Module: Frequently Asked Questions
What is AI detection?
Deteksi AI mengacu pada identifikasi konten yang dihasilkan oleh sistem kecerdasan buatan, yang membedakannya dari konten yang ditulis oleh manusia.
Bagaimana ZeroGPT mendeteksi teks yang dihasilkan AI?
ZeroGPT menggunakan model dan algoritme yang sudah terlatih untuk menganalisis pola teks dan mengidentifikasi konten yang dihasilkan oleh AI.
Apa saja batasan dari ZeroGPT?
ZeroGPT Keterbatasannya termasuk kurangnya pemahaman kontekstual, ketidakmampuan untuk mendeteksi pola-pola yang tidak biasa, dan terlalu bergantung pada data yang sudah terlatih.
Apa sajakah beberapa alternatif untuk ZeroGPT untuk deteksi AI?
Alternatif lain termasuk alat yang menggunakan pembelajaran transfer, model pembelajaran ensemble, jaringan saraf grafik, dan teknik pembelajaran aktif.
How can transfer learning improve AI detection accuracy?
Transfer learning meningkatkan akurasi dengan menyempurnakan model yang telah dilatih sebelumnya dengan dataset tertentu, meningkatkan kemampuannya untuk mendeteksi pola-pola yang berbeda.
What is ensemble learning?
Ensemble learning combines multiple algorithms to improve detection accuracy, leveraging the strengths of different models.
How do graph neural networks help in AI detection?
Graph neural networks map relationships within text, providing deeper insights and improving detection accuracy by analyzing text structure.
What is active learning in AI detection?
Active learning involves continuously updating the model with new data, improving its accuracy over time by adapting to new patterns.
Why is data preprocessing important in AI detection?
Data preprocessing cleans the data and extracts relevant features, enhancing model performance and detection accuracy.
What are the future trends in AI detection?
Future trends include advancements in NLP, integration with AI ethics and compliance, and development of real-time detection systems.
Conclusion: Advancing AI Detection Beyond ZeroGPT
Enhancing AI detection accuracy requires addressing the limitations of existing tools like ZeroGPT. By adopting alternative approaches such as transfer learning, ensemble learning models, graph neural networks, and active learning techniques, we can improve detection capabilities. Implementing these solutions with proper data preprocessing, model selection, and regular evaluation ensures robust AI detection systems. Future trends in NLP advancements and real-time detection systems will further advance AI detection accuracy, promoting transparency and accountability in AI-generated content detection.
For the best tool to use, consider Detektor AI Online, which offers a range of features and high accuracy.